A Step-by-Step Walkthrough of the Wilcoxon Rank Sum Test and Mann-Whitney U Test
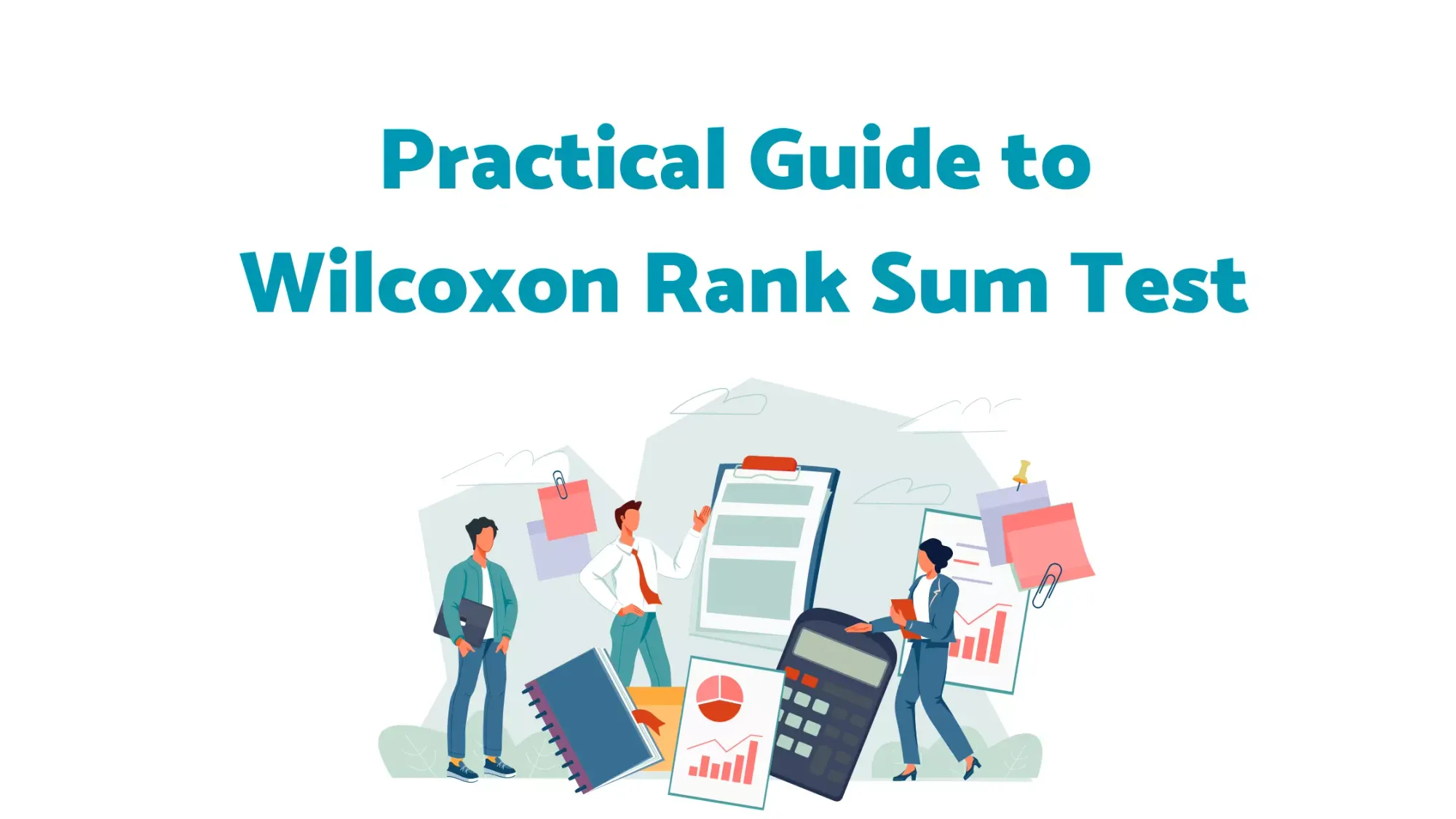
In the world of statistics and data analysis, understanding the nature of your data and choosing the appropriate test is paramount. While many of us are introduced to the t-test as a standard method for comparing group means, it's not always the best fit, especially when dealing with non-normally distributed data or ordinal scales. Herein lies the importance of the Wilcoxon Rank Sum Test, a non-parametric test that often proves to be a robust alternative.
The Wilcoxon Rank Sum Test, frequently referred to as the Mann-Whitney U test, offers a solution for those tricky datasets that don't quite fit the bill for a t-test. Whether you're grappling with skewed data, ordinal responses, or simply want a test that doesn't assume a specific data distribution, the Wilcoxon Rank Sum Test is an invaluable tool. This guide aims to demystify this test, exploring its intricacies and offering practical examples to solidify your grasp.
The Wilcoxon Rank Sum Test, due to its non-parametric nature, is particularly useful in scenarios where the assumptions of traditional parametric tests, such as the t-test, are violated.
What is the Wilcoxon Rank Sum Test?
The Wilcoxon Rank Sum Test , which is sometimes called the Mann-Whitney U test, is a non-parametric statistical test used to determine if there is a significant difference between two independent groups when the data is not normally distributed or when dealing with ordinal variables. This test is a handy alternative when the assumptions of the t-test, like normality, are violated.
The Wilcoxon Rank Sum Test works by ranking all the data points from both groups together, from the smallest to the largest. Once ranked, the test then examines the sum of the ranks from each group. If the two groups come from identical populations, then the rank sums should be roughly equal. However, if one group consistently has higher or lower ranks than the other, this indicates a significant difference between the groups.
Example 1: Comparing Efficacy of Two Medications
Suppose a pharmaceutical company wants to compare the efficacy of two pain relief medications: Drug A and Drug B. They collect data on the level of pain relief (on a scale of 1 to 10, with 10 being complete pain relief) experienced by patients using each drug. The data might look something like this:
Patient | Drug A | Drug B |
---|---|---|
1 | 7 | 8 |
2 | 6 | 9 |
3 | 7 | 8 |
4 | 6 | 9 |
5 | 8 | 7 |
Since pain relief scores are ordinal and the data may not be normally distributed, the Wilcoxon Rank Sum Test can be used to determine if one drug provides significantly better pain relief than the other.
Example 2: Assessing Job Satisfaction
Imagine a company that wants to assess job satisfaction between two departments: Sales and Engineering. Employees from both departments are asked to rank their job satisfaction on a scale from 1 (least satisfied) to 5 (most satisfied). The data might look as follows:
Employee | Sales | Engineering |
---|---|---|
A | 3 | 4 |
B | 4 | 3 |
C | 2 | 3 |
D | 3 | 4 |
E | 4 | 4 |
Again, since job satisfaction scores are ordinal and might not be normally distributed, the Wilcoxon Rank Sum Test would be an appropriate method to determine if there's a significant difference in job satisfaction between the two departments.
In both examples, the test would rank all the scores, sum the ranks for each group, and then compare these sums to determine if there is a statistically significant difference between the groups.
When to Use the Test?
The Wilcoxon Rank Sum Test (or the Mann-Whitney U Test), due to its non-parametric nature, is particularly useful in scenarios where the assumptions of traditional parametric tests, such as the t-test, are violated. Here are some key scenarios where the Wilcoxon Rank Sum Test is applicable:
- Non-Normal Data: One of the primary reasons to use the Wilcoxon Rank Sum Test is when the data does not follow a normal distribution. Many statistical tests assume normality, and violating this assumption can lead to inaccurate conclusions.
- Ordinal Data: The test is ideal for data that can be ranked. For example, survey responses that use a Likert scale (e.g., Strongly Disagree, Disagree, Neutral, Agree, Strongly Agree) are ordinal in nature.
- Independent Groups: The two groups being compared must be independent of each other. This means that the observations in one group should not influence the observations in the other group.
Examples:
Scenario 1: A researcher is comparing the effectiveness of two therapies, A and B, for reducing anxiety. Participants rank their level of anxiety relief on a scale from 1 (no relief) to 5 (complete relief). Given that the data is ordinal, the Wilcoxon Rank Sum Test would be appropriate.
Scenario 2: A study is conducted to compare the growth of plants in two different types of soil. However, upon data collection, it's evident that the growth measurements are not normally distributed. Instead of a t-test, the Wilcoxon Rank Sum Test would be more suitable.
When NOT to Use the Test?
While the Wilcoxon Rank Sum Test is versatile, it's not always the best choice. Here are instances where other tests might be more suitable:
- Normally Distributed Data with Equal Variances: If the data is normally distributed and the variances of the two groups are equal, a standard two-sample t-test is more powerful and provides more precise results.
- Dependent or Paired Groups: If you have paired data (i.e., measurements are taken on the same subjects under different conditions), the Wilcoxon Signed-Rank Test, not the Rank Sum Test, would be appropriate.
- More than Two Groups: If comparing more than two independent groups, the Kruskal-Wallis Test, another non-parametric method, should be used instead.
Examples:
Scenario 1: A company is comparing the average salaries of two different job positions, and the salary data for both positions are normally distributed with equal variances. In this case, a two-sample t-test would be more appropriate.
Scenario 2: A researcher measures blood pressure in patients before and after administering a particular drug. Since the measurements are paired (taken on the same individuals), the Wilcoxon Signed-Rank Test, not the Rank Sum Test, would be the correct choice.
While the Wilcoxon Rank Sum Test is a powerful tool, always ensure that its assumptions and conditions align with your specific dataset and research question.
The Definition and Mathematical Background
The fundamental idea behind the test is to rank all data points from both groups together, from the smallest to the largest value. Once all values are ranked, the test examines the sum of the ranks from each group. If the two groups come from identical populations, then we'd expect the rank sums for both groups to be roughly equal. Significant deviations from this expectation can indicate differences between the groups.
Formulating the Test:
Given two samples:
- Group A of size \( n \)
- Group B of size \( m \)
- Ranking the Data:
- Combine all observations from both groups.
- Rank the observations from the smallest to the largest. If there are ties (i.e., identical observations), assign them the average of the ranks they span.
- Calculating Rank Sums:
- Calculate the sum of the ranks for Group A, denoted as \( R_A \).
- Similarly, calculate the sum of the ranks for Group B, denoted as \( R_B \).
- Calculating the Test Statistic:
\[
U_A = n \times m + \frac{n(n + 1)}{2} - R_A
\]
\[
U_B = R_B - n \times m
\]- The test statistic, \( U \), can be calculated using the rank sums. There are two equivalent expressions for \( U \), one based on Group A and the other on Group B:
- The smaller of \( U_A \) and \( U_B \) is usually taken as the test statistic \( U \).
- Expectation and Variance:
\[
E(U) = \frac{n \times m}{2}
\]
\[
\text{Var}(U) = \frac{n \times m \times (n + m + 1)}{12}
\]- Under the null hypothesis (i.e., both groups come from the same population), the expected value of \( U \) is:
- The variance of \( U \) is:
- Significance Testing:
\[
Z = \frac{U - E(U)}{\sqrt{\text{Var}(U)}}
\]- Under the null hypothesis, and with large enough sample sizes, \( U \) is approximately normally distributed. This property allows us to standardize \( U \) and compare it to a standard normal distribution to determine its significance. The standardized test statistic, \( Z \), is given by:
- The value of \( Z \) can then be used to determine the p-value and test the hypothesis.
Interpretation:
- If the value of \( U \) is much smaller or much larger than its expected value under the null hypothesis, this suggests that the two groups differ.
- A significant p-value (typically < 0.05) indicates that the distributions of the two groups are not the same.
In practice, many software packages and statistical tools handle these calculations and provide the p-value directly, making it easy to interpret the results of the test.
Example: Comparing Exam Scores
Imagine two teachers, Mr. A and Ms. B, who want to determine if their teaching methods result in different exam scores for their students. They collect scores from a recent exam:
- Mr. A's Class: 85, 90, 78, 92, 88
- Ms. B's Class: 80, 82, 88, 85, 91
Rank All Scores:
Combine all scores and rank them:
78 (1), 80 (2), 82 (3), 85 (4.5), 85 (4.5), 88 (6.5), 88 (6.5), 90 (8), 91 (9), 92 (10)
(Note: For tied ranks, we assign the average of the ranks. Here, 85 and 88 are tied.)
Calculate Rank Sums:
- Mr. A's Class Rank Sum: 1 + 8 + 6.5 + 10 + 6.5 = 32
- Ms. B's Class Rank Sum: 2 + 3 + 4.5 + 4.5 + 9 = 23
Calculate U Statistic:
Using the formula:
\[ U_A = n \times m + \frac{n(n + 1)}{2} - R_A \]
Where \( n \) and \( m \) are the sizes of the two groups. Here, both \( n \) and \( m \) are 5.
\[ U_A = 5 \times 5 + \frac{5(5 + 1)}{2} - 32 \]
\[ U_A = 25 + 15 - 32 \]
\[ U_A = 8 \]
Similarly, \( U_B \) can be calculated and will equal 17, but we generally take the smaller \( U \) value, so \( U = 8 \).
Determine Significance:
For this small sample size, you would typically consult a Wilcoxon Rank Sum Test table to determine significance or use statistical software to get the p-value.
Practical Examples
Through these examples, we aim to illuminate the process and rationale behind the test, offering a comprehensive grasp of its utility in empirical research.
Example 1: Comparing Two Teaching Methods
Imagine we conducted a survey in which students were asked to rank their satisfaction with two teaching methods, A and B, on a scale from 1 (least satisfied) to 5 (most satisfied). The results are as follows:
Student | Method A | Method B |
---|---|---|
1 | 3 | 4 |
2 | 4 | 5 |
3 | 2 | 3 |
4 | 3 | 3 |
5 | 4 | 4 |
Given this ordinal data, we can use the Wilcoxon Rank Sum Test to determine if there's a significant difference in student satisfaction between the two teaching methods.
Example 2: Analyzing Customer Satisfaction
A company wants to understand the customer satisfaction of its two products: X and Y. Customers ranked their satisfaction on a scale from 1 (least satisfied) to 10 (most satisfied).
Customer | Product X | Product Y |
---|---|---|
A | 6 | 7 |
B | 5 | 8 |
C | 7 | 6 |
D | 6 | 5 |
E | 8 | 9 |
Using the Wilcoxon Rank Sum Test, the company can determine if there's a statistically significant difference in customer satisfaction between products X and Y.
The Fundamental Question
At its core, the test seeks to answer a simple question: When we randomly pick one observation from each group, how often is the observation from one group larger than the observation from the other group?
Intuition Behind Rankings
The brilliance of the Wilcoxon Rank Sum Test lies in its approach. Instead of directly comparing raw data values, it relies on the ranks of these values. This is why it's a "rank sum" test. Ranking data has a few key advantages:
- It's Resilient to Outliers: Extreme values can heavily influence many statistical tests. By ranking data, we essentially standardize it, making the test less sensitive to outliers.
- It Handles Non-Normal Data: Many tests assume data is normally distributed. The Wilcoxon Rank Sum Test doesn't. By using ranks, it can handle skewed data, making it a non-parametric test.
The Essence of the U Statistic
The "U" in the Mann-Whitney U Test stands for the number of "unfavorable" comparisons. In other words, if you were to randomly select a value from each group, the U statistic represents how often a value from the first group is smaller than a value from the second group.
The intuition here is straightforward: If the two groups are similar, we'd expect the number of times a value from Group A exceeds a value from Group B to be roughly equal to the number of times a value from Group B exceeds a value from Group A. If these counts differ significantly, it suggests a difference between the groups.
Visual Analogy
Imagine you have two buckets of marbles, one representing each group. Each marble is labeled with a data value. Now, if you were to randomly draw one marble from each bucket and compare the numbers, you'd want to know: How often does the marble from the first bucket have a higher number than the one from the second bucket?
If it's about half the time, the groups are probably similar. But if the marble from one bucket consistently has a higher (or lower) value, it suggests a difference between the two buckets.
The beauty of the Wilcoxon Rank Sum Test lies in its simplicity. By converting data into ranks and focusing on the relative comparisons between two groups, it offers a robust and intuitive way to gauge differences, especially when traditional assumptions about data don't hold.
Practical Use Cases
The Wilcoxon Rank Sum Test, given its versatility as a non-parametric method, can find applications across many fields and disciplines. Here's a list of potential applications in various fields:
Medicine & Healthcare:
- Drug Efficacy: Comparing the effectiveness of two different drugs or treatments based on patient outcomes or symptom relief scores.
- Therapy Evaluation: Assessing the effectiveness of two different therapeutic techniques based on patient-reported improvement scales.
- Diagnosis Tools: Comparing the accuracy or speed of two diagnostic tools based on ordinal grading.
Agriculture:
- Fertilizer Testing: Evaluating the yield or health of crops under two different fertilizers.
- Pest Control: Comparing the effectiveness of two pest control methods based on damage scores or pest counts.
- Growth Conditions: Assessing plant growth or health under two different environmental conditions, such as light intensity or soil type.
Business & Economics:
- Product Testing: Comparing customer satisfaction or feedback scores for two product variants.
- Website Design: Evaluating user engagement or conversion rates between two website designs or layouts.
- Employee Satisfaction: Comparing job satisfaction levels between two departments or under two different management styles.
Environmental Science:
- Conservation Techniques: Assessing the success of two conservation methods based on wildlife population counts or health metrics.
- Pollution Control: Comparing the efficacy of two pollution control strategies based on pollution metrics or environmental health indicators.
Social Sciences:
- Educational Techniques: Evaluating student performance or feedback under two different teaching methodologies or curricula.
- Survey Analysis: Analyzing public opinion or behavior based on responses to two different campaigns or interventions.
- Psychological Interventions: Assessing the impact of two different interventions or techniques on mental health or well-being metrics.
Technology & Computer Science:
- Algorithm Comparison: Comparing the performance or accuracy of two algorithms based on ordinal efficiency grades.
- User Experience (UX): Evaluating user satisfaction or ease of use between two software interfaces or application designs.
- Hardware Testing: Comparing the performance or reliability scores of two pieces of hardware or components.
Sports & Exercise Science:
- Training Regimens: Comparing athlete performance or health metrics under two different training routines or diets.
- Equipment Evaluation: Assessing player feedback or performance metrics using two different pieces of sports equipment.
- Recovery Methods: Evaluating athlete recovery or injury metrics under two different recovery techniques or treatments.
Any field or discipline that requires the comparison of two independent groups, especially when data is ordinal or non-normally distributed, can potentially benefit from the Wilcoxon Rank Sum Test.
Where does the name come from?
The names associated with these statistical tests are derived from the statisticians who developed and popularized them:
- Wilcoxon Rank Sum Test: This test is named after Frank Wilcoxon, an American chemist and statistician. He introduced this test, along with another related test (the Wilcoxon Signed-Rank Test for paired data), in a 1945 paper. The "Rank Sum" in the name reflects the methodology of the test, which involves ranking combined data from two groups and then summing and comparing the ranks.
- Mann-Whitney U Test: This alternative name for the test comes from Henry B. Mann and Donald R. Whitney, two statisticians who independently formulated a test based on the same principles as Wilcoxon's around the same time in the 1940s. The "U" in the name refers to the test statistic calculated using the rank sums, which measures the degree of difference between the two groups.
It's worth noting that, while the methods proposed by Wilcoxon and by Mann and Whitney were developed independently and might have slight variations in their formulations, they are equivalent in their application and results. As a result, the names "Wilcoxon Rank Sum Test" and "Mann-Whitney U Test" are often used interchangeably in the literature.
Implementation in popular statistical tools
The Wilcoxon Rank Sum Test, given its widespread applicability, is supported by many popular statistical and mathematical software packages and programming languages. Below is a brief overview of how the test is implemented in some of these:
R
In R, the wilcox.test()
function from the base package can be used to conduct the Wilcoxon Rank Sum Test.
# Data for two groups
group1 <- c(5, 7, 8, 9, 10)
group2 <- c(3, 4, 6, 7, 8)
# Conduct the test
wilcox.test(group1, group2)
Reference: R Documentation. wilcox.test
Python (with SciPy):
In Python, the mannwhitneyu()
function from the scipy.stats
module performs this test.
from scipy.stats import mannwhitneyu
# Data for two groups
group1 = [5, 7, 8, 9, 10]
group2 = [3, 4, 6, 7, 8]
# Conduct the test
stat, p = mannwhitneyu(group1, group2)
print('Statistic:', stat, 'P-value:', p)
Reference: SciPy mannwhitneyu
SPSS:
In SPSS:
- Go to the
Analyze
menu. - Choose
Nonparametric Tests
. - Select
Independent Samples...
. - Place your dependent variable into the
Test Variable List
box and your grouping variable into theGrouping Variable
box. - Click on
Define Groups
and specify the groups. - Check
Mann-Whitney U
underTest Type
. - Click
OK
.
MATLAB:
In MATLAB, the ranksum()
function can be used.
% Data for two groups
group1 = [5, 7, 8, 9, 10];
group2 = [3, 4, 6, 7, 8];
% Conduct the test
[p, h, stats] = ranksum(group1, group2);
Reference: MathWorks. ranksum
SAS:
In SAS, you can use the NPAR1WAY
procedure with the WILCOXON
option.
PROC NPAR1WAY DATA=mydata WILCOXON;
CLASS group;
VAR score;
RUN;
Reference: SAS Documentation. The NPAR1WAY Procedure
Stata:
In Stata, use the ranksum
command.
ranksum score, by(group)
In all these tools, the test will provide a test statistic and a p-value. The p-value can be used to determine if there's a significant difference between the two groups. If the p-value is less than a chosen significance level (e.g., 0.05), then the difference is considered statistically significant.
Reference: Stata Manual. ranksum
Conclusion
The Wilcoxon Rank Sum Test offers a versatile and robust method for comparing two independent groups, especially when the data is non-normally distributed or ordinal. By understanding when and how to apply this test, researchers and analysts can derive more accurate insights from their data.
Remember, while the Wilcoxon Rank Sum Test is a powerful tool, always ensure that it's the right test for your specific scenario. It's equally crucial to interpret the results in the context of the research question and the nature of the data.